3 Conic quadratic optimization¶
This chapter extends the notion of linear optimization with quadratic cones. Conic quadratic optimization, also known as second-order cone optimization, is a straightforward generalization of linear optimization, in the sense that we optimize a linear function under linear (in)equalities with some variables belonging to one or more (rotated) quadratic cones. We discuss the basic concept of quadratic cones, and demonstrate the surprisingly large flexibility of conic quadratic modeling.
3.1 Cones¶
Since this is the first place where we introduce a non-linear cone, it seems suitable to make our most important definition:
A set \(K\subseteq\real^n\) is called a convex cone if
for every \(x,y\in K\) we have \(x+y\in K\),
for every \(x\in K\) and \(\alpha\geq 0\) we have \(\alpha x\in K\).
For example a linear subspace of \(\real^n\), the positive orthant \(\real_{\geq 0}^n\) or any ray (half-line) starting at the origin are examples of convex cones. We leave it for the reader to check that the intersection of convex cones is a convex cone; this property enables us to assemble complicated optimization models from individual conic bricks.
3.1.1 Quadratic cones¶
We define the \(n\)-dimensional quadratic cone as
The geometric interpretation of a quadratic (or second-order) cone is shown in Fig. 3.1 for a cone with three variables, and illustrates how the boundary of the cone resembles an ice-cream cone. The 1-dimensional quadratic cone simply states nonnegativity \(x_1\geq 0\).
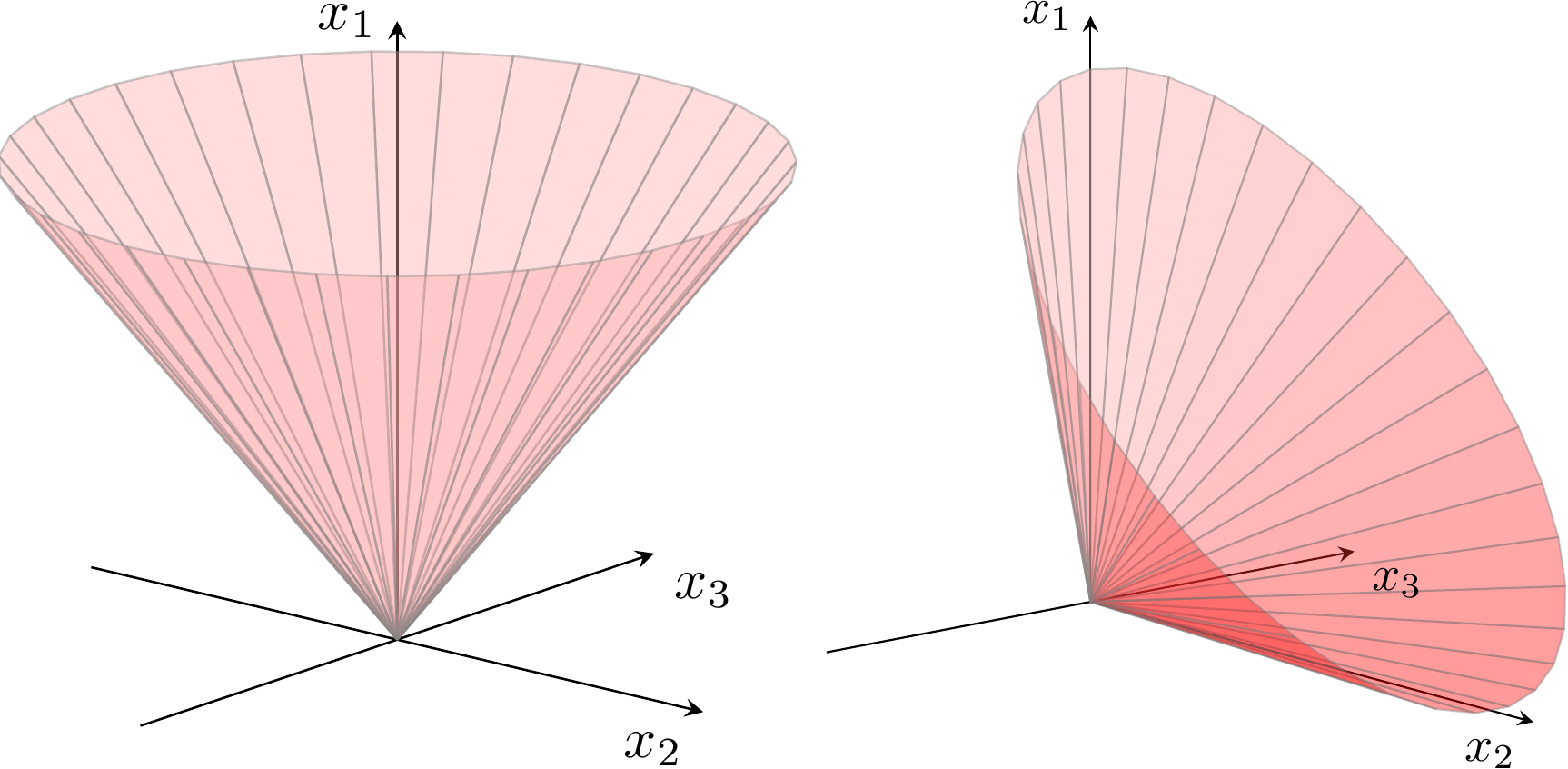
Fig. 3.1 Boundary of quadratic cone \(x_1\geq \sqrt{x_2^2+x_3^2}\) and rotated quadratic cone \(2x_1x_2\geq x_3^2\), \(x_1,x_2\geq 0\).¶
3.1.2 Rotated quadratic cones¶
An \(n-\)dimensional rotated quadratic cone is defined as
As the name indicates, there is a simple relationship between quadratic and rotated quadratic cones. Define an orthogonal transformation
Then it is easy to verify that
and since \(T\) is orthogonal we call \(\Q_r^n\) a rotated cone; the transformation corresponds to a rotation of \(\pi/4\) in the \((x_1,x_2)\) plane. For example if \(x\in \Q^3\) and
then
and similarly we see that
Thus, one could argue that we only need quadratic cones \(\Q^n\), but there are many examples where using an explicit rotated quadratic cone \(\Q^n_r\) is more natural, as we will see next.
3.2 Conic quadratic modeling¶
In the following we describe several convex sets that can be modeled using conic quadratic formulations or, as we call them, are conic quadratic representable.
3.2.1 Absolute values¶
In Sec. 2.2.2 (Absolute value) we saw how to model \(|x|\leq t\) using two linear inequalities, but in fact the epigraph of the absolute value is just the definition of a two-dimensional quadratic cone, i.e.,
3.2.2 Euclidean norms¶
The Euclidean norm of \(x\in \R^n\),
essentially defines the quadratic cone, i.e.,
The epigraph of the squared Euclidean norm can be described as the intersection of a rotated quadratic cone with an affine hyperplane,
3.2.3 Convex quadratic sets¶
Assume \(Q \in \R^{n \times n}\) is a symmetric positive semidefinite matrix. The convex inequality
may be rewritten as
Since \(Q\) is symmetric positive semidefinite the epigraph
is a convex set and there exists a matrix \(F\in \R^{k\times n}\) such that
(see Sec. 6 (Semidefinite optimization) for properties of semidefinite matrices). For instance \(F\) could be the Cholesky factorization of \(Q\). Then
and we have an equivalent characterization of (3.5) as
Frequently \(Q\) has the structure
where \(I\) is the identity matrix, so
and hence
is a conic quadratic representation of (3.5) in this case.
3.2.4 Second-order cones¶
A second-order cone is occasionally specified as
where \(A \in \R^{m\times n}\) and \(c \in \R^n\). The formulation (3.7) is simply
or equivalently
As will be explained in Sec. 8 (Duality in conic optimization), we refer to (3.8) as the dual form and (3.9) as the primal form. An alternative characterization of (3.7) is
which shows that certain quadratic inequalities are conic quadratic representable.
3.2.5 Simple sets involving power functions¶
Some power-like inequalities are conic quadratic representable, even though it need not be obvious at first glance. For example, we have
or in a similar fashion
For a more complicated example, consider the constraint
This is equivalent to a statement involving two cones and an extra variable
because
In practice power-like inequalities representable with similar tricks can often be expressed much more naturally using the power cone (see Sec. 4 (The power cones)), so we will not dwell on these examples much longer.
3.2.6 Rational functions¶
A general non-degenerate rational function of one variable \(f(x)=\frac{ax+b}{gx+h}\) can always be written in the form \(f(x)=p+\frac{q}{gx+h}\), and when \(q>0\) it is convex on the set where \(gx+h>0\). The conic quadratic model of
with variables \(t,x\) can be written as:
We can generalize it to a quadratic-over-linear function \(f(x)=\frac{ax^2+bx+c}{gx+h}\). By performing long polynomial division we can write it as \(f(x)=rx+s+\frac{q}{gx+h}\), and as before it is convex when \(q>0\) on the set where \(gx+h>0\). In particular
can be written as
In both cases the argument boils down to the observation that the target function is a sum of an affine expression and the inverse of a positive affine expression, see Sec. 3.2.5 (Simple sets involving power functions).
3.2.7 Harmonic mean¶
Consider next the hypograph of the harmonic mean,
It is not obvious that the inequality defines a convex set, nor that it is conic quadratic representable. However, we can rewrite it in the form
which suggests the conic representation:
Alternatively, in case \(n=2\), the hypograph of the harmonic mean can be represented by a single quadratic cone:
As this representation generalizes the harmonic mean to all points \(x_1 + x_2 \geq 0\), as implied by (3.12), the variable bounds \(x\geq 0\) and \(t\geq 0\) have to be added seperately if so desired.
3.2.8 Quadratic forms with one negative eigenvalue¶
Assume that \(A \in \R^{n \times n}\) is a symmetric matrix with exactly one negative eigenvalue, i.e., \(A\) has a spectral factorization (i.e., eigenvalue decomposition)
where \(Q^T Q = I\), \(\Lambda=\Diag(-\alpha_1, \alpha_2, \dots, \alpha_n)\), \(\alpha_i \geq 0\). Then
is equivalent to
This shows \(x^T A x \leq 0\) to be a union of two convex subsets, each representable by a quadratic cone. For example, assuming \(q_1^T x \geq 0\), we can characterize (3.13) as
3.2.9 Ellipsoidal sets¶
The set
describes an ellipsoid centred at \(c\). It has a natural conic quadratic representation, i.e., \(x\in {\cal E}\) if and only if
3.3 Conic quadratic case studies¶
3.3.1 Quadratically constrained quadratic optimization¶
A general convex quadratically constrained quadratic optimization problem can be written as
where all \(Q_i\in\real^{n\times n}\) are symmetric positive semidefinite. Let
where \(F_i \in \R^{k_i \times n}\). Using the formulations in Sec. 3.2.3 (Convex quadratic sets) we then get an equivalent conic quadratic problem
Assume next that \(k_i\), the number of rows in \(F_i\), is small compared to \(n\). Storing \(Q_i\) requires about \(n^2/2\) space whereas storing \(F_i\) then only requires \(nk_i\) space. Moreover, the amount of work required to evaluate \(x^T Q_i x\) is proportional to \(n^2\) whereas the work required to evaluate \(x^T F_i^T F_ i x = \| F_i x \|^2\) is proportional to \(nk_i\) only. In other words, if \(Q_i\) have low rank, then (3.16) will require much less space and time to solve than (3.15). We will study the reformulation (3.16) in much more detail in Sec. 10 (Quadratic optimization).
3.3.2 Robust optimization with ellipsoidal uncertainties¶
Often in robust optimization some of the parameters in the model are assumed to be unknown exactly, but there is a simple set describing the uncertainty. For example, for a standard linear optimization problem we may wish to find a robust solution for all objective vectors \(c\) in an ellipsoid
A common approach is then to optimize for the worst-case scenario for \(c\), so we get a robust version
The worst-case objective can be evaluated as
where we used that \(\sup_{\| u \|_2\leq 1} v^T u = (v^T v)/\|v\|_2=\|v\|_2\). Thus the robust problem (3.17) is equivalent to
which can be posed as a conic quadratic problem
3.3.3 Markowitz portfolio optimization¶
In classical Markowitz portfolio optimization we consider investment in \(n\) stocks or assets held over a period of time. Let \(x_i\) denote the amount we invest in asset \(i\), and assume a stochastic model where the return of the assets is a random variable \(r\) with known mean
and covariance
The return of our investment is also a random variable \(y = r^Tx\) with mean (or expected return)
and variance (or risk)
We then wish to rebalance our portfolio to achieve a compromise between risk and expected return, e.g., we can maximize the expected return given an upper bound \(\gamma\) on the tolerable risk and a constraint that our total investment is fixed,
Suppose we factor \(\Sigma = GG^T\) (e.g., using a Cholesky or a eigenvalue decomposition). We then get a conic formulation
In practice both the average return and covariance are estimated using historical data. A recent trend is then to formulate a robust version of the portfolio optimization problem to combat the inherent uncertainty in those estimates, e.g., we can constrain \(\mu\) to an ellipsoidal uncertainty set as in Sec. 3.3.2 (Robust optimization with ellipsoidal uncertainties).
It is also common that the data for a portfolio optimization problem is already given in the form of a factor model \(\Sigma=F^TF\) of \(\Sigma=I+F^TF\) and a conic quadratic formulation as in Sec. 3.3.1 (Quadratically constrained quadratic optimization) is most natural. For more details see Sec. 10.3 (Example: Factor model).
3.3.4 Maximizing the Sharpe ratio¶
Continuing the previous example, the Sharpe ratio defines an efficiency metric of a portfolio as the expected return per unit risk, i.e.,
where \(r_f\) denotes the return of a risk-free asset. We assume that there is a portfolio with \(\mu^T x>r_f\), so maximizing the Sharpe ratio is equivalent to minimizing \(1/S(x)\). In other words, we have the following problem
The objective has the same nature as a quotient of two affine functions we studied in Sec. 2.2.5 (Homogenization). We reformulate the problem in a similar way, introducing a scalar variable \(z\geq 0\) and a variable transformation
Since a positive \(z\) can be chosen arbitrarily and \((\mu - r_fe)^T x>0\), we can without loss of generality assume that
Thus, we obtain the following conic problem for maximizing the Sharpe ratio,
and we recover \(x = y/z\).
3.3.5 A resource constrained production and inventory problem¶
The resource constrained production and inventory problem [Zie82] can be formulated as follows:
where \(n\) denotes the number of items to be produced, \(b\) denotes the amount of common resource, and \(r_j\) is the consumption of the limited resource to produce one unit of item \(j\). The objective function represents inventory and ordering costs. Let \(c_j^p\) denote the holding cost per unit of product \(j\) and \(c_j^r\) denote the rate of holding costs, respectively. Further, let
so that
is the average holding costs for product \(j\). If \(D_j\) denotes the total demand for product \(j\) and \(c_j^o\) the ordering cost per order of product \(j\) then let
and hence
is the average ordering costs for product \(j\). In summary, the problem finds the optimal batch size such that the inventory and ordering cost are minimized while satisfying the constraints on the common resource. Given \(d_j, e_j \geq 0\) problem (3.21) is equivalent to the conic quadratic problem
It is not always possible to produce a fractional number of items. In such case \(x_j\) should be constrained to be integers. See Sec. 9 (Mixed integer optimization).