11.5 Logistic regression¶
Logistic regression is an example of a binary classifier, where the output takes one two values 0 or 1 for each data point. We call the two values classes.
Formulation as an optimization problem
Define the sigmoid function
Next, given an observation
The weights vector
When training a logistic regression algorithm we are given a sequence of training examples
Of course every single
The training problem with regularization (a standard technique to prevent overfitting) is now equivalent to
This can equivalently be phrased as
Implementation
As can be seen from (11.20) the key point is to implement the softplus bound
and further to
# t >= log( 1 + exp(u) ) coordinatewise
def softplus(M, t, u):
n = t.getShape()[0]
z1 = M.variable(n)
z2 = M.variable(n)
M.set(z1 + z2 == 1,
Expr.hstack(z1, Expr.constTerm(n, 1.0), u-t) == Domain.inPExpCone(),
Expr.hstack(z2, Expr.constTerm(n, 1.0), -t) == Domain.inPExpCone())
Once we have this subroutine, it is easy to implement a function that builds the regularized loss function model (11.20).
# Model logistic regression (regularized with full 2-norm of theta)
# X - n x d matrix of data points
# y - length n vector classifying training points
# lamb - regularization parameter
def logisticRegression(X, y, lamb=1.0):
n, d = int(X.shape[0]), int(X.shape[1]) # num samples, dimension
M = Model()
theta = M.variable(d)
t = M.variable(n)
reg = M.variable()
M.objective(ObjectiveSense.Minimize, Expr.sum(t) + lamb * reg)
M.constraint(Var.vstack(reg, theta), Domain.inQCone())
signs = list(map(lambda y: -1.0 if y==1 else 1.0, y))
softplus(M, t, Expr.mulElm(X @ theta, signs))
return M, theta
Example: 2D dataset fitting
In the next figure we apply logistic regression to the training set of 2D points taken from the example ex2data2.txt . The two-dimensional dataset was converted into a feature vector
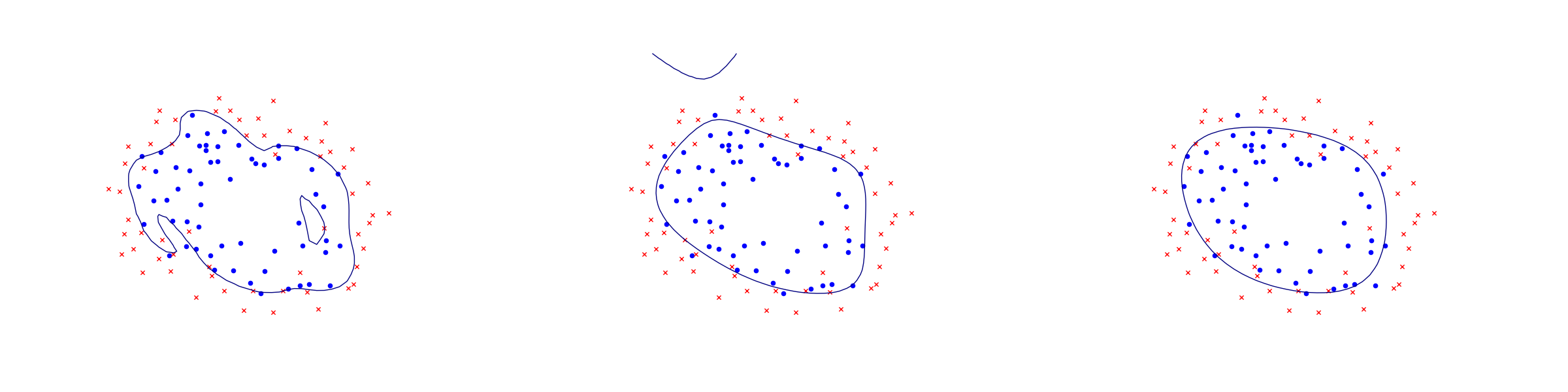
Fig. 11.6 Logistic regression example with none, medium and strong regularization (small, medium, large