11.2 Logistic regression¶
Logistic regression is an example of a binary classifier, where the output takes one two values 0 or 1 for each data point. We call the two values classes.
Formulation as an optimization problem
Define the sigmoid function
Next, given an observation \(x\in\real^d\) and a weights \(\theta\in\real^d\) we set
The weights vector \(\theta\) is part of the setup of the classifier. The expression \(h_\theta(x)\) is interpreted as the probability that \(x\) belongs to class 1. When asked to classify \(x\) the returned answer is
When training a logistic regression algorithm we are given a sequence of training examples \(x_i\), each labelled with its class \(y_i\in \{0,1\}\) and we seek to find the weights \(\theta\) which maximize the likelihood function
Of course every single \(y_i\) equals 0 or 1, so just one factor appears in the product for each training data point. By taking logarithms we can define the logistic loss function:
The training problem with regularization (a standard technique to prevent overfitting) is now equivalent to
This can equivalently be phrased as
Implementation
As can be seen from (11.13) the key point is to implement the softplus bound \(t\geq \log(1+e^u)\), which is the simplest example of a log-sum-exp constraint for two terms. Here \(t\) is a scalar variable and \(u\) will be the affine expression of the form \(\pm \theta^Tx_i\). This is equivalent to
and further to
This formulation can be entered using affine conic constraints (see Sec. 6.2 (From Linear to Conic Optimization)).
// Adds ACCs for t_i >= log ( 1 + exp((1-2*y[i]) * theta' * X[i]) )
// Adds auxiliary variables, AFE rows and constraints
public static void softplus(Task task, int d, int n, int theta, int t, double[,] X, bool[] y)
{
int nvar = task.getnumvar();
int ncon = task.getnumcon();
long nafe = task.getnumafe();
task.appendvars(2*n); // z1, z2
task.appendcons(n); // z1 + z2 = 1
task.appendafes(4*n); //theta * X[i] - t[i], -t[i], z1[i], z2[i]
int z1 = nvar, z2 = nvar+n;
int zcon = ncon;
long thetaafe = nafe, tafe = nafe+n, z1afe = nafe+2*n, z2afe = nafe+3*n;
int k = 0;
// Linear constraints
int[] subi = new int[2*n];
int[] subj = new int[2*n];
double[] aval = new double[2*n];
for(int i = 0; i < n; i++)
{
// z1 + z2 = 1
subi[k] = zcon+i; subj[k] = z1+i; aval[k] = 1; k++;
subi[k] = zcon+i; subj[k] = z2+i; aval[k] = 1; k++;
}
task.putaijlist(subi, subj, aval);
task.putconboundsliceconst(zcon, zcon+n, boundkey.fx, 1, 1);
task.putvarboundsliceconst(nvar, nvar+2*n, boundkey.fr, -inf, inf);
// Affine conic expressions
long[] afeidx = new long[d*n+4*n];
int[] varidx = new int[d*n+4*n];
double[] fval = new double[d*n+4*n];
k = 0;
// Thetas
for(int i = 0; i < n; i++) {
for(int j = 0; j < d; j++) {
afeidx[k] = thetaafe + i; varidx[k] = theta + j;
fval[k] = ((y[i]) ? -1 : 1) * X[i,j];
k++;
}
}
// -t[i]
for(int i = 0; i < n; i++) {
afeidx[k] = thetaafe + i; varidx[k] = t + i; fval[k] = -1; k++;
afeidx[k] = tafe + i; varidx[k] = t + i; fval[k] = -1; k++;
}
// z1, z2
for(int i = 0; i < n; i++) {
afeidx[k] = z1afe + i; varidx[k] = z1 + i; fval[k] = 1; k++;
afeidx[k] = z2afe + i; varidx[k] = z2 + i; fval[k] = 1; k++;
}
// Add the expressions
task.putafefentrylist(afeidx, varidx, fval);
// Add a single row with the constant expression "1.0"
long oneafe = task.getnumafe();
task.appendafes(1);
task.putafeg(oneafe, 1.0);
// Add an exponential cone domain
long expdomain = task.appendprimalexpconedomain();
// Conic constraints
for(int i = 0; i < n; i++)
{
task.appendacc(expdomain, new long[]{z1afe+i, oneafe, thetaafe+i}, null);
task.appendacc(expdomain, new long[]{z2afe+i, oneafe, tafe+i}, null);
}
}
Once we have this subroutine, it is easy to implement a function that builds the regularized loss function model (11.13).
// Model logistic regression (regularized with full 2-norm of theta)
// X - n x d matrix of data points
// y - length n vector classifying training points
// lamb - regularization parameter
public static double[] logisticRegression(Env env,
double[,] X,
bool[] y,
double lamb)
{
int n = X.GetLength(0);
int d = X.GetLength(1); // num samples, dimension
using (Task task = new Task(env, 0, 0))
{
// Variables [r; theta; t]
int nvar = 1+d+n;
task.appendvars(nvar);
task.putvarboundsliceconst(0, nvar, boundkey.fr, -inf, inf);
int r = 0, theta = 1, t = 1+d;
// Objective lambda*r + sum(t)
task.putobjsense(mosek.objsense.minimize);
task.putcj(r, lamb);
for(int i = 0; i < n; i++)
task.putcj(t+i, 1.0);
// Softplus function constraints
softplus(task, d, n, theta, t, X, y);
// Regularization
// Append a sequence of linear expressions (r, theta) to F
long numafe = task.getnumafe();
task.appendafes(1+d);
task.putafefentry(numafe, r, 1.0);
for(int i = 0; i < d; i++)
task.putafefentry(numafe + i + 1, theta + i, 1.0);
// Add the constraint
task.appendaccseq(task.appendquadraticconedomain(1+d), numafe, null);
// Solution
task.optimize();
return task.getxxslice(soltype.itr, theta, theta+d);
}
}
Example: 2D dataset fitting
In the next figure we apply logistic regression to the training set of 2D points taken from the example ex2data2.txt . The two-dimensional dataset was converted into a feature vector \(x\in\real^{28}\) using monomial coordinates of degrees at most 6.
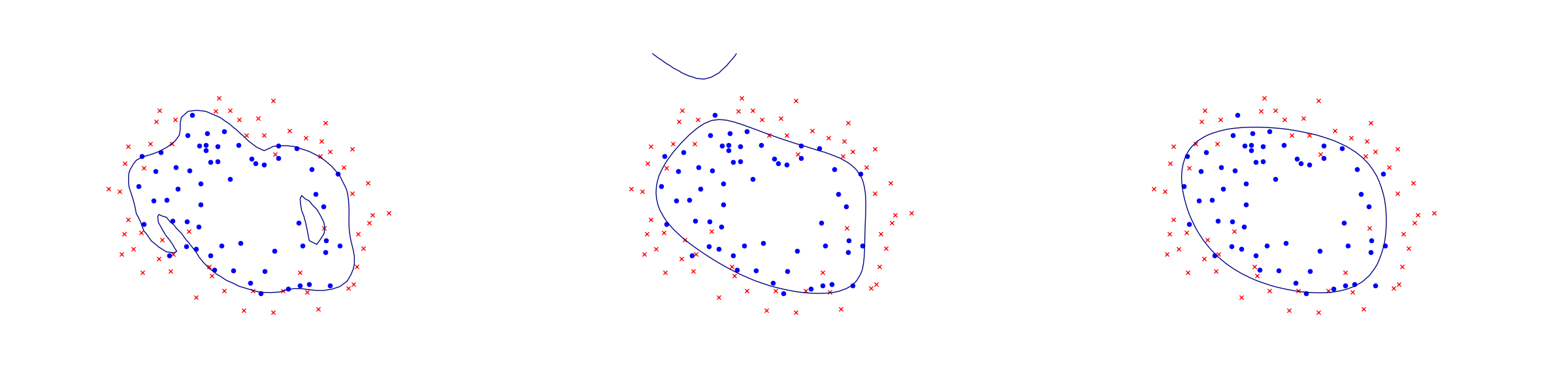
Fig. 11.2 Logistic regression example with none, medium and strong regularization (small, medium, large \(\lambda\)). Without regularization we get obvious overfitting.¶